May 2019 | 1144 words | 4-minute read
Amazon started personalizing our shopping experience twenty years ago. Now, Netflix creates our own home theatre – experimenting even to the extent of changing billboard images. For instance, if you watch Doctor Strange and The Imitation Game, it offers the next Cumberbatch movie; no surprises there. However, what is surprising is that it may change the poster of Twelve Years a Slave from showing Chiwetel Ejiofor (the lead) to showing Benedict Cumberbatch (a minor role as William Ford), just so you will pick it up.
You may indeed be happy to watch a movie you never knew had Cumberbatch in it, and Netflix sold you a new genre of content. Digital businesses are trying to do special things for hundreds of millions of customers and over millions of products, over each interaction. The segment of one has arrived.
Powering the Segment of One
The power behind the segment of one lies in predictive analytics powered by Big Data technologies. These work with large volumes of transactional and operational data containing a mix of structured and unstructured content. Predictive analysis helps in identifying different ways to keep a customer engaged on a site. Knowledge and insights extracted from an analysis of a customer’s past history, including his or her browsing history, past transactions, items abandoned from their carts, buying behavior, etc., are combined with data tracked during the current visit to generate recommendations and targeted offers. Adding profile information obtained either from customer relationship management (CRM) systems, gathered through surveys, or customer-provided feedback is often found to significantly improve the results.
Despite strides in personalization, it is far from a perfect thing. As personalization becomes more widespread, the many complexities of dealing with a many-headed monster are coming to the forefront, which is likely to keep researchers in the field of Computational Intelligence busy for quite some time to come.
Micro-Segmentation
With humongous computational resources at their disposal, eCommerce establishments are taking personalization to the logical extreme, where micro-segmentation culminates at the “group of one”. It is also possible to do powerful long-tail analysis, which takes into account seemingly unpopular but niche and novel products that interest only specific customer segments.
Historically, business analysts tried to segment customers into groups based on similar behavioral patterns exhibited by each group. This is still a valid grouping. Micro-segmentation aims at finding small groups of customers, who exhibit fine-grained behavioral similarities based on historical actions and current actions gathered through real-time tracking. Coupled with customer profile data and ample details about available inventory, predictive analytics models built for each of these segments can provide right inputs for targeted marketing to optimize the nuanced customer experience. These models can also generate context-sensitive next best actions or offers.
High-Performance Computing and Hyper-Personalization
The dream of hyper-personalization can be attained only if it is coupled with a delivery platform that can track millions of users and predict their next needs or actions based on past history. High-performance computing practices aggregate computing power to deliver application performances that are not possible to be delivered otherwise over typical workstations. Building application-agnostic hyper-personalization platforms that can adapt to multiple recommendation engines and domains while scaling up robustly is a research challenge.
Role-based Personalization in the Enterprise
Digital-born retail has been the poster child for personalization. But for businesses with global footprints, their enterprises, and their extensions (vendor, partners, supply chains, customers), information overload has created the need for personalized content delivery.
Contextually intelligent systems fuse multi-structured information gathered from heterogeneous sources (structured and/or unstructured) in real-time to predict the next likely action of the user, and thereby, optimize the uptake possibility of the recommended content, not just for a single application but for a multitude of applications.
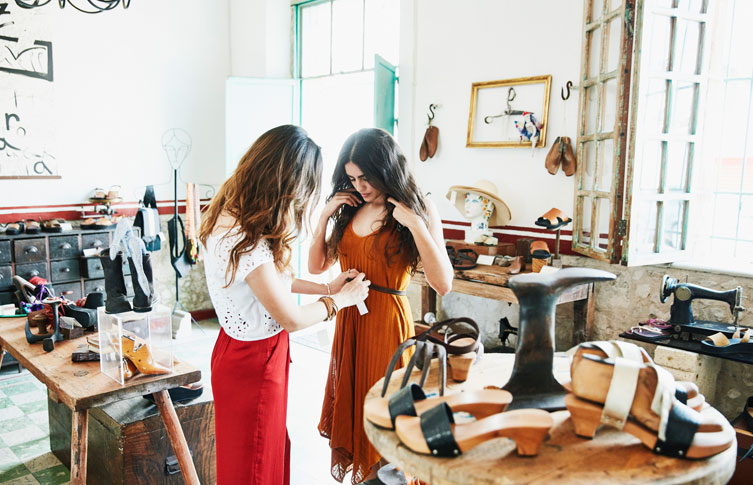
Personalization – Future Challenges
While the recommendation models discussed here appear to be fairly generic in design, their applications across all fields have not seen the same level of success as eRetail. The primary reason for this is the lack of mature behavioral models for other domains. Though the challenges for each area appear to be different from the others, there are some common problems that personalized recommenders are trying to address.
- Handling Novelty – Though repetition and frequency derived from personal preferences drive a bulk of personalized recommendation, sectors like travel and hospitality or entertainment that have seen a huge surge in the number of online transactions in their areas have seen the limited success of personalization to date. A major reason for this is the incapability of the current models to incorporate novelty as a user preference. Novel or unique elements, which have no history of being chosen or more importantly, are not even similar to products chosen earlier, cannot be accommodated easily in the predictive model. However, novelty is a key factor that drives customer choices for these sectors. This is referred to as the cold-start problem in the science of recommendation. Determining the novelty of an item is comparatively easier than determining which novel items to recommend to whom, how, and when. These are some of the major challenges that personalization systems are trying to deal with.
- Objective-driven Information Recommendation – Recommending information or textual content to users face another type of challenge. Due to the explosive growth in the amount of available digital information, filtering systems that can detect the right information for a user are in great demand. Along with user preferences that can be learned from their past behavior, personalization in this case also has to determine the intangible quantity that can measure the worth of a piece of information in the user’s personal and professional life. While role-based information filtering systems are being designed, the challenge lies in formally specifying the concepts of roles and responsibilities and integrating them to create an objective-driven information-filtering framework.
- Security and Privacy Concerns – The recent success of personalization in many sectors can be attributed to the data that a user shares with content providers voluntarily and involuntarily. With rising concerns over privacy and security breaches, research in the area of personalization will have to deal with this seriously. An immediate point of concern is the enforcement of the new EU-wide privacy rules in the form of General Data Protection Regulation (GDPR) that is expected to create a seismic shift not only in the way personal information is defined but also how it is collected, processed, used, and transferred. Several key data elements like sexual orientation, organizational memberships, and so on, will require the ‘explicit consent’ of users before they can be used. An important technological aspect that would be affected by the introduction of GDPR is the regulation on using ‘persistent online identifiers’ such as cookies, which are small text files storing tiny pieces of data on a user’s browser. What it would mean for personalization platforms, which store these identifiers, will have to be seen since violators of GDPR provisions are likely to face huge penalties.
Lead investigator Dr Lipika Dey is a Chief Scientist at TCS Research and Innovation. Dr Rekha Singhal, TCS, has worked with both corporate and academic research.
Reproduced from "Reimagining Research". You can download the full PDF of this paper from here.